Active Interpretation of Disparate Alternatives (AIDA)
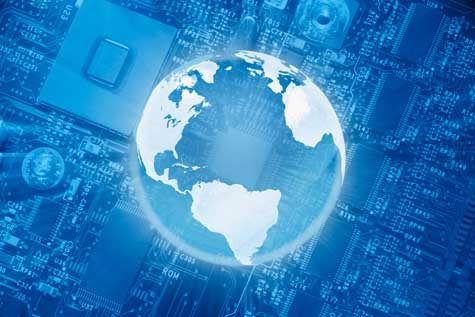
Dr. Wil Corvey
The United States Government has an interest in developing and maintaining a strategic understanding of events, situations, and trends around the world, in a variety of domains. The information used in developing this understanding comes from many disparate sources, in a variety of genres, and data types, and as a mixture of structured and unstructured data. Unstructured data can include text or speech in English and a variety of other languages, as well as images, videos, and other sensor information. Even structured sources can vary in the expressiveness, semantics, and specificity of their representations. Moreover, analysis is complicated by the need to overcome the noisy, conflicting, and potentially intentionally deceptive nature of the data.
It is a challenge for those who strive to achieve and maintain an understanding of these events, situations, and trends that information from each medium is often analyzed independently, without the context provided by information from other media. Often, each independent analysis results in only one interpretation, with alternatives being eliminated due to lack of evidence, even in the absence of contradictory evidence. When these independent, impoverished analyses are combined, generally late in the analysis process, the result can be a single apparent consensus view that does not reflect a true consensus.
The goal of Active Interpretation of Disparate Alternatives (AIDA) is to develop a multihypothesis semantic engine that generates explicit alternative interpretations of events, situations, and trends from a variety of unstructured sources, for use in noisy, conflicting, and potentially deceptive information environments. This engine must be capable of mapping knowledge elements automatically derived from multiple media sources into a common semantic representation, aggregating information derived from those sources, and generating and exploring multiple hypotheses about the events, situations, and trends of interest. This engine must establish confidence measures for the derived knowledge and hypotheses, based on the accuracy of the analysis and the coherence of the semantic representation of each hypothesis. This engine must also be capable of utilizing knowledge in the common semantic representation and the generated hypotheses as alternate contexts for the media analysis algorithms by altering their models or prior probabilities to enhance accuracy and resolve ambiguities in line with expectations from the context. In addition, the engine must be able to communicate with its user to reveal the generated hypotheses and to allow the user to alter the hypotheses or to suggest new ones.